Google’s AI advertising revolution: More privacy, but problems remain
April 1, 2021
Share
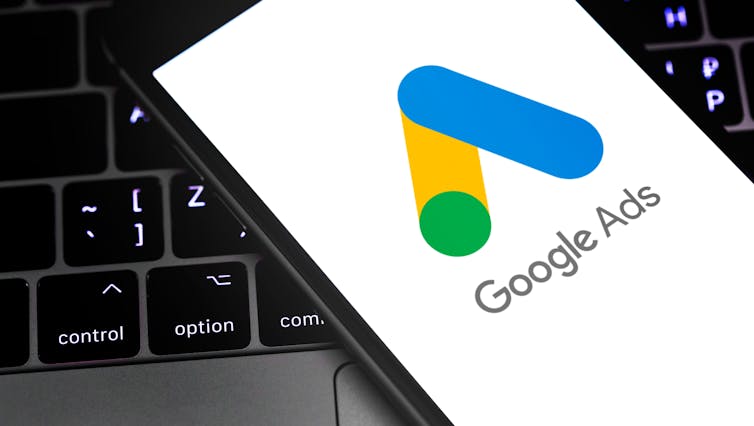
In March 2021, Google announced that it was ending support for third-party cookies, and moving to “.” Even though the move was expected within the industry and by academics, there is still confusion about the new model, and cynicism about whether it truly constitutes the kind of revolution in online privacy that Google claims.
To assess this, we need to understand this new model and what is changing. The current advertising technology (adtech) approach is one in which platform corporations give us a “free” service in exchange for our data. The data is collected via third-party cookies downloaded to our devices, that allow a browser to record our internet activity. This is used to create profiles and predict our susceptibility to specific ad campaigns.
Recent advances have allowed digital advertisers to use deep learning, a form of artificial intelligence (AI) wherein humans do not set the parameters. Although more powerful, this is still consistent with the old model, relying on collecting and storing our data to train models and make predictions. Google’s plans go further still.
Patents and plans
All corporations have their , and Google is more secretive than most. However, patents can reveal some of what they’re up to. After an exploration of Google patents, we found U.S. patent , “Targeted advertising using temporal analysis of user-specific data”: a patent for a system that predicts the effectiveness of ads based on a user’s “temporal data,” snapshots of what a user is doing at a specific point instead of indiscriminate mass data collection over a longer time period.
We can also make inferences by examining work from other organizations. Research funded by adtech company Bidtellect demonstrated that . They used deep learning to model users’ interests from temporal data.
Alongside contextual advertising — which displays ads based on the content of the website on which they appear — this could lead to more privacy-conscious advertising. And without storing personally identifiable information, this approach would be compliant with progressive laws like the European Union’s General Data Protection Regulation (GDPR).
Google has also released some information through the , a set of public proposals to restructure adtech. At its core are , a decentralized AI system deployed by the latest browsers. , federated learning differs from traditional machine learning techniques that collect and process data centrally. Instead, a deep learning model is downloaded temporarily onto a device, where it trains on our data, before returning to the server as an updated model to be combined with others.
With FLoCs, the deep learning model will be downloaded to Google Chrome browsers, and analyze local browser data. It then sorts the user into a “cohort,” a group of a few thousand users sharing a set of traits identified by the model. It makes an encrypted copy of itself, deletes the original and sends the encrypted copy back to Google, leaving behind only a cohort number. Since each cohort contains thousands of users, Google maintains that the individual becomes virtually unidentifiable.
Cohorts and concerns
In this new model, advertisers don’t select individual characteristics to target, but instead advertise to a given cohort, . Although FLoCs may sound less effective than collecting our individual data, they realize “95 per cent of the conversions per dollar spent when compared with cookie-based advertising.”
The bidding process for ads will also take place on the browser, using another system .” Soon, Google adtech will all work this way, contained on a web browser, making constant ad predictions based on our most recent actions, without collecting or storing personally identifiable information.
We see three key concerns. First, this is only part of a much larger AI picture Google is building across the internet. Through , for example, Google continues to use data gained from individual website-based first-person cookies to train machine learning models and potentially build individual profiles.
Secondly, does it matter how an organization comes to “know” us? Or is it the fact that it knows? Google is giving us back legally acceptable individual data privacy, however it is intensifying its ability to know us and commodify our online activity. Is privacy the right to control our individual data, or for the essence of ourselves to remain unknown without consent?
The final issue concerns AI. The limitations, biases and injustice around AI are now . We need to understand how deep learning tools in FLoCs group us into cohorts, attribute qualities to cohorts and what those qualities represent. Otherwise, , FLoCs could further entrench socio-economic inequalities and divisions.
___________________________________________________________________________
, Associate Professor in Sociology, and , Masters student, .
This article is republished from under a Creative Commons license. Read the .
The Conversation is seeking new academic contributors. Researchers wishing to write articles should contact Melinda Knox, Associate Director, Research Profile and Initiatives, at knoxm@queensu.ca.